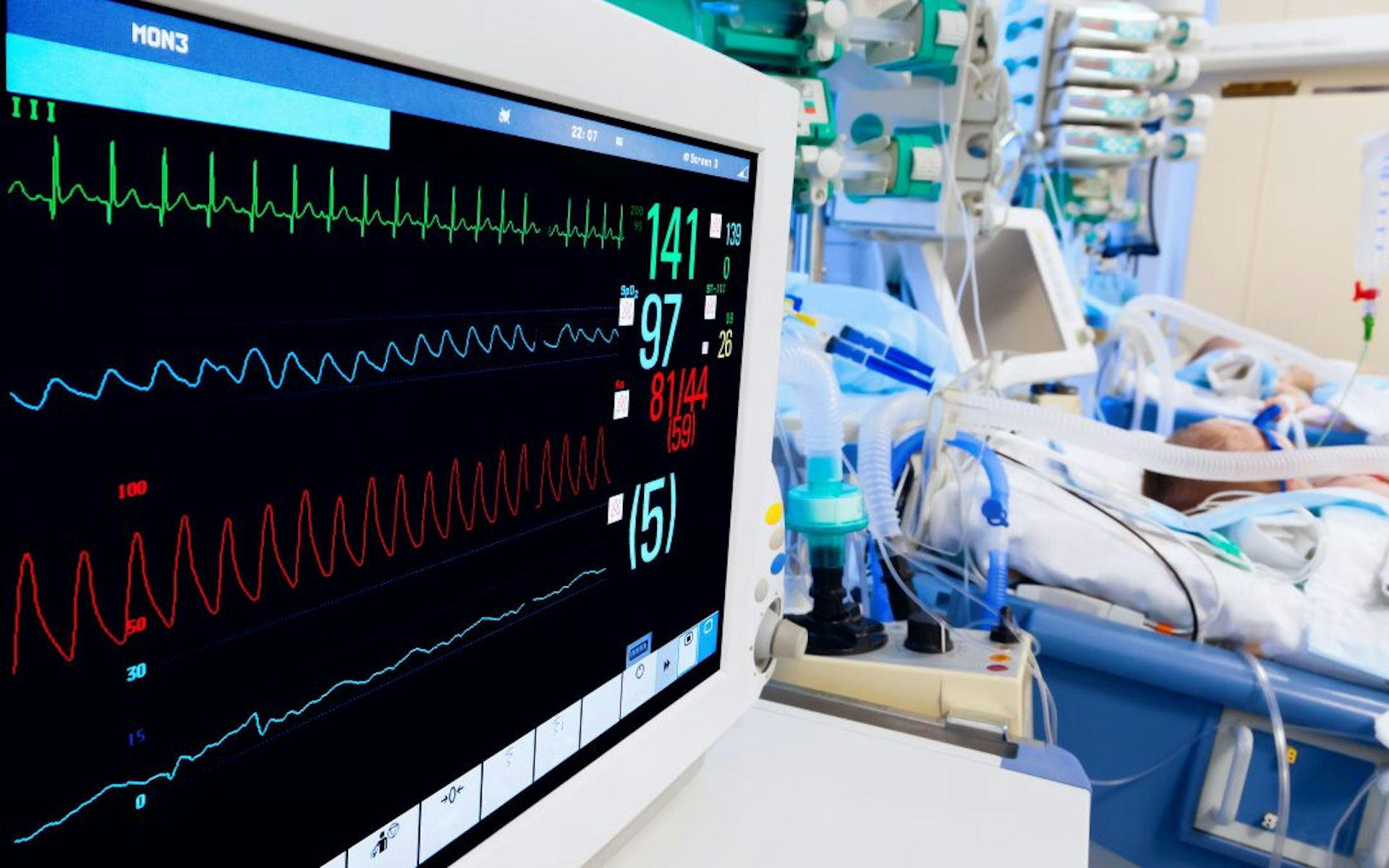
Imagine it’s 2030 – and a typical day in a Nigerian healthcare setting. In earlier decades, when a patient walked in, they could see piles of folders and a clutter of pens scattered all over the office. They’d have a long wait before being seen by a medical professional. Today, clinicians use technology to navigate easily through a system that’s centred on the patient.
So what has changed? Information in physical folders and files has been captured and used. A connected healthcare system has become a reality, driven by machine learning. Machine learning is basically getting a computer programme to perform a task without giving it explicit instructions.
Thanks to machine learning, patient care in 2030 has changed for good. Machine learning can now look at complex data to identify patterns and make timely predictions about the onset of disease and clinical outcomes. It does so by aggregating the huge amount of information from clinical notes, pathology results, sensor readings and medical images.
For decades, 97% of the data in these sources was unused, trapped in stacks of paper. In 2030, the Nigerian healthcare system can deliver proactive, predictive healthcare that is widely accessible and affordable.
Pattern recognition algorithms that help detect hepatitis B virus in vulnerable Nigerian populations are a step in this direction.
This work forms the basis of my PhD. Working closely with clinicians and medical providers at the Nigerian Institute of Medical Research and the University of Ilorin Teaching Hospital, our team is conducting a machine learning study at the Australian National University. We aim to improve access to affordable testing and care for the millions who are unaware of their hepatitis B status.
Predictive care
Nigeria’s rapidly growing population is overstretching the understaffed and underfunded healthcare system. But machine learning and predictive analytics could help in three areas: expanding access, improving quality of care, and reducing costs.
For Nigeria and similar countries, hepatitis B virus provides an example. Hepatitis B is a leading cause of chronic liver disease and death worldwide. At least 1 in 10 Nigerians live with viral hepatitis B, translating into about 20 million infected people. Yet they are missing from the global public health agenda because of the cost and other limitations of diagnostic tests. Most people living with this silent killer are unaware of their infection status, and at risk of transmitting the virus to others.
Machine learning can improve screening, access to treatment and the integration of prevention and care in the Nigerian healthcare system. My supervisors, Brett Lidbury and Alice Richardson, earlier proposed a novel pattern recognition bioinformatics system to replace mouse models of human diseases.
I am building on their work to investigate patterns in routine blood tests and clinical data. These patterns are being used to develop a model which allows the prediction of hepatitis B infection in Nigerian patients. Once validated, this may allow early detection of infection without needing expensive tests.
The goal is that by 2030, Nigerian healthcare will use an intelligent system that can identify patterns in a patient’s clinical data, use the patterns to indicate early on whether the patient has been infected with hepatitis B virus, and link them to care before their condition becomes worse. Success will mean that rates of infection, liver disease and liver cancer decline through timely interventions.
Back to our present reality
In 2021, the system still uses traditional paper records. It’s ill-equipped with technological infrastructure and lacks quality electronic health data. This impedes connected care.
Further, many Nigerian health facilities are unable to spend their budget on research to facilitate predictive care. Adopting machine learning is unfortunately not going to be an easy ride, as the system has been structured in a way that makes it difficult to influence a real change.
Still, we see clear signs that a machine learning-enabled system can one day become a reality in Nigeria. There have already been a few pilots and test cases. For example, a Nigerian start-up is using a signal processing and machine learning-enabled system to improve the diagnosis of birth asphyxia in low-resource settings.
The journey to a connected healthcare system in Nigeria is long, and the government cannot do it alone. Healthcare centres, public health stakeholders and private industries must work with the Nigerian government to ensure that machine learning systems are fully inter-operable, transparent, and devoid of bias and inequality. Standard ethical guidelines that protect the way in which machine learning uses patient data will also become an urgent priority.
The most powerful use of machine learning is to enhance human capabilities, and not replace them. The heart of connected care isn’t just about new technologies, it’s about people – the people who need to be cared for and the people who work to deliver personalised care.
Busayo Ajuwon, Research Associate |PhD Candidate, Australian National University; Alice Richardson, Associate professor, Australian National University, and Brett Lidbury, Associate Professor
This article is republished from The Conversation under a Creative Commons license.